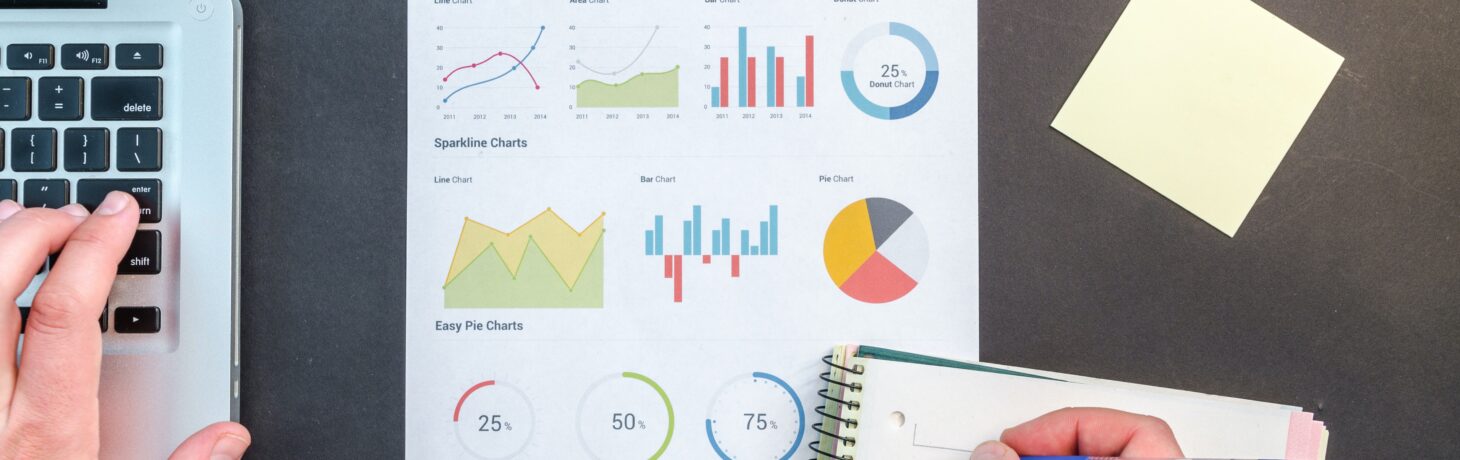
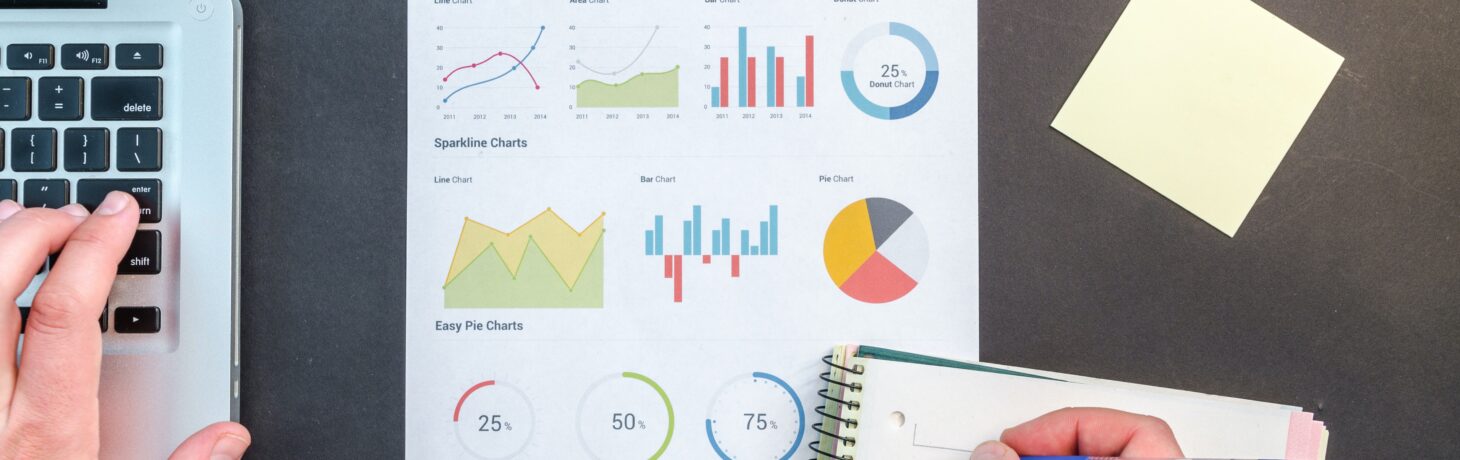
insights
7 trends in clinical trial data
Seven Trends in Clinical Trial Data goes in-depth on one of our most discussed topics during CORE 2017. We asked CORE sponsor, Medidata, to share their thoughts on this developing subject. For more, check out Behind closed doors at CORE 2017.
If you haven’t noticed, we have entered an unprecedented era of big data, where the world’s data volume doubles every two years! For instance, global wearable device traffic is expected to surpass 40 zettabytes by 2020…one zettabyte equals 1 trillion gigabytes. And this big data explosion is just as apparent within clinical trials. Non-CRF (case report reform) data now represents 70% of clinical data and is growing at 40% per year.
It’s hard to understate the potential change this can bring to clinical development, but how do you extract value from all this new data?
Clinical trials are incorporating data from so many more sources today: genomic sequencing, medical imaging data, lab data, wearables, and other mHealth data. And beyond clinical data, trial sponsors need to be able to incorporate operational data, financial data and real-world evidence to get the most out of their R&D investments. Today’s clinical research requires a technology platform that can ingest and integrate data from any data source. Otherwise, this data is at risk of being underutilized.
At Medidata, we see sponsors and clinical research organization (CRO) partners transforming the way they think about study planning and study conduct.
We organized a forum of Medidata sponsors and partners, consisting of representatives from many of the largest pharmaceutical, biotech, device and CRO companies, to gather invaluable insight on the priorities, drivers, and trends shaping clinical development. We recently asked the group to identify key trends that are impacting clinical trials. As a group, they identified seven areas that should be familiar to all in clinical development:
- Increasingly complex trials: More and more interest is building in iteratively modifying parameters of a trial protocol based on real-time outcomes
- Precision medicine: Precision medicine is on the rise as the technology increases to administer therapeutics to patients in highly individualized ways.
- Virtual trials: Virtual clinical trials represent a new method of collecting safety and efficacy data from clinical trial participants through the use of mobile health (mHealth) technology without requiring them to visit facilities or doctor’s offices.
- Patient centricity: Patients are no longer recipients of healthcare, they are active participants. Patient centricity requires more than simply gaining insight from patients; it includes a focus on meeting patient needs and translating patient insights to products that improve outcomes.
- Big data analytics and AI modeling: Advances in data analytics and visualization enable researchers to explore and interact with large-scale, often aggregate, bodies of data.
- Ever-more data: As the genomics revolution hits the mainstream and real-world data (such as wearable sensors and patient social media trails) becomes accessible and accepted, clinical trials have an explosion of data to ingest, manage and analyze.
- Blockchain: Blockchain is a linked data structure that establishes a timestamped, chronological record of history that cannot be tampered with or deleted because no single person or organization has control over the ledger.
With these growing trends come various data challenges throughout study planning, startup, and conduct. Within our group of sponsors and CROs, it became clear that sophisticated data analytics are needed to analyze cross-sponsor data pools to overcome the new challenges of the day.
For one, researchers need to standardize genomic data for clinical data packages, especially if supporting global clinical trial programs. Until recently, integrating genomic data into trial data repositories was a clumsy process. Medidata Clinical Trial Genomics (CTG), which was published for the 2017 Annual Meeting of the American Society of Clinical Oncology (ASCO), automates integration between genomics data and central trial data pools. It then applies machine learning algorithms to link the genomic data to the phenotypic data to provide the analytical results needed in a trial.
There are also opportunities to mine clinical data assets for earlier insight during clinical development. If the advent of genomic sequencing allows researchers to look at trials at the cellular level, historical data gives researchers a look at trials from a much grander scale.
Organizations like Medidata are building platforms to bring together disparate data sets from trials of similar indications to improve understanding. With its database of 3.8 million patient data from more than 13,000 clinical trials, Medidata Synthetic Control Arm (SCA) creates control groups, precisely matched at the subject level for a trial’s inclusion/exclusion criteria—minimizing site and study-specific bias. SCA offers the possibility of exploratory subgroup analysis, improves the selection of compounds for next phase and the design of subsequent trials, and can reduce failure rates in Phase II and III studies.
Luckily, as data assets and sources increase, so do the technology solutions to support them. In Medidata’s case, we started as an EDC company. But because of the complex and changing nature of R&D, we have created a data-enabled, unified platform to be able to ingest, standardize, store and analyze data from all sources. We started as a software company, but we’re now a data company because extracting value from clinical data is at the core improving clinical trial performance.